Maxime Agostini
Traditionally, companies have relied upon data masking, sometimes called de-identification, to protect data privacy. The basic idea is to remove all personally identifiable information (PII) from each record. However, a number of high-profile incidents have shown that even supposedly de-identified data can leak consumer privacy.
In 1996, an MIT researcher identified the then-governor of Massachusetts’ health records in a supposedly masked dataset by matching health records with public voter registration data. In 2006, UT Austin researchers re-identifed movies watched by thousands of individuals in a supposedly anonymous dataset that Netflix had made public by combining it with data from IMDB.
In a 2022 Nature article, researchers used AI to fingerprint and re-identify more than half of the mobile phone records in a supposedly anonymous dataset. These examples all highlight how “side” information can be leveraged by attackers to re-identify supposedly masked data.
These failures led to differential privacy. Instead of sharing data, companies would share data processing results combined with random noise. The noise level is set so that the output does not tell a would-be attacker anything statistically significant about a target: The same output could have come from a database with the target or from the exact same database but without the target. The shared data processing results do not disclose information about anybody, hence preserving privacy for everybody.
Operationalizing differential privacy was a significant challenge in the early days. The first applications were primarily the provenance of organizations with large data science and engineering teams like Apple, Google or Microsoft. As the technology becomes more mature and its cost decreases, how can all organizations with modern data infrastructures leverage differential privacy in real-life applications?
Differential privacy applies to both aggregates and row-level data
When the analyst cannot access the data, it is common to use differential privacy to produce differentially private aggregates. The sensitive data is accessible through an API that only outputs privacy-preserving noisy results. This API may perform aggregations on the whole dataset, from simple SQL queries to complex machine learning training tasks.
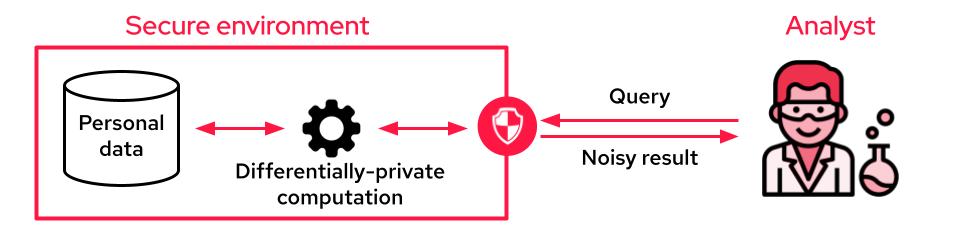
One of the disadvantages of this setup is that, unlike data masking techniques, analysts no longer see individual records to “get a feel for the data.” One way to mitigate this limitation is to provide differentially private synthetic data where the data owner produces fake data that mimics the statistical properties of the original dataset.
The whole process is done with differential privacy to guarantee user privacy. Insights on synthetic data are generally noisier than when running aggregate queries, so it is not advisable to perform aggregations from synthetic data; when possible, differentially private aggregation on the raw data is preferable.
However, synthetic data does provide analysts with the look and feel to which they are accustomed. Data professionals can now have access to their row-level data while eating their differential privacy cake.
Choosing the right architecture
The way data is separated from the analyst depends on where the data is stored. The two main architectures are global differential privacy and local differential privacy.
In the global setup, a central party aggregates the data from many individuals and processes it with differential privacy. This is what the U.S. Census Bureau did in 2022 when it released census data for the entire country’s population.
In the local setup, the data remains on the user’s device; queries from data practitioners are processed on the device with differential privacy. This is being used by Google in Chrome or Apple on the iPhone, for instance.
While more secure, the local approach requires significantly more noise per user, making it unsuitable for small user bases. It is also much harder to deploy since every computation needs to be orchestrated across thousands or millions of independent user devices.
Most enterprises have already collected raw user data on their servers where data can be processed. For them, global differential privacy is the right approach.
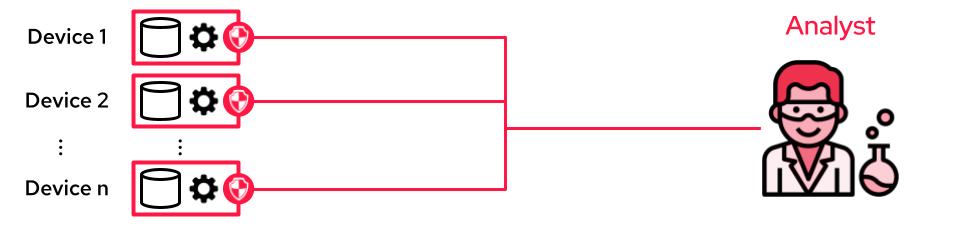
Data sharing with differential privacy
Differential privacy can also be used for data sharing. Obviously, one should not expect to share a differentially private dataset and still be able to match records. It would be a blatant breach of the promise of privacy (differential privacy actually guarantees this will never be possible). This leaves the practitioner with two options.
The first option is to share differentially private synthetic data. However, while synthetic datasets can be trained to be accurate on a predetermined set of queries or metrics, they come with no accuracy guarantees for new queries outside of the predetermined set. For this reason, insights built on synthetic data can be risky to use for decision-making. Plus, matching users is clearly impossible on a purely synthetic dataset.
The second option is to share access to the confidential data via a differentially private aggregation mechanism instead of the data itself. The insights are typically much more accurate than if estimated from differentially private synthetic data. Even better, matching individuals remains possible.
The analyst may submit a query that first joins their new outside user-level data with the confidential user-level data, run the query on the joint data with differential privacy, and derive truly anonymous insights from it. This approach achieves the best of both worlds: optimal privacy while preserving the full granularity of each record.
It makes one assumption, though: While the original confidential information remains confidential, the new outside data must travel onto a third-party system during computation. This approach has been popularized by Google and Facebook under the name of data clean rooms.
There are variations that rely on cryptographic techniques to protect the confidentiality of both datasets that are beyond the scope of this article.
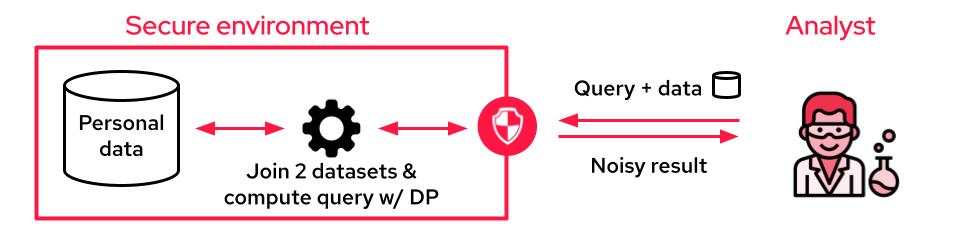
Proprietary and open source software
To implement differential privacy, one should not start from scratch, as any implementation mistake could be catastrophic for the privacy guarantees. On the open source side, there are plenty of libraries that provide the basic differential privacy building blocks.
The main ones are:
- OpenDP (SmartNoise Core and SmartNoise SDK): an initiative led by Harvard University providing differentially private primitives and tools to transform any SQL query into a differentially private one.
- Google DP and TensorFlow Privacy: Google open source project providing primitives for analytics and machine learning.
- OpenMined PyDP: a Python library built on top of Google DP.
OpenMined PipelineDP: also built on top of Google DP’s privacy on Beam. - Pytorch Opacus: a Facebook project for differentially private deep learning in pytorch.
- PyVacy: from Chris Waites.
- IBM Diffprivlib: an IBM initiative with differential privacy primitives and machine learning models.
However, just like having access to a secure cryptographic algorithm is not sufficient to build a secure application, having access to secure implementation of differential privacy is not sufficient to power a data lake with privacy guarantees.
Some startups are bridging this gap by proposing proprietary solutions available off the shelf.
Leapyear has raised $53 million to propose differentially private processing of databases. Tonic raised $45 million to let developers build and test software with fake data generated with the same mathematical protection.
Gretel, with $68 million in the bank, uses differential privacy to propose privacy engineering as a service while open sourcing some of the synthetic data generation models. Privitar, a data privacy solution that has raised $150 million so far, now features differential privacy among other privacy techniques.
Datafleets was developing distributed data analysis powered by differential privacy before being acquired by LiveRamp for over $68 million. Sarus, where one of the authors is a co-founder, recently joined Y Combinator and proposes to power data warehouses with differential privacy without changing existing workflows.
In conclusion
Differential privacy doesn’t just better protect privacy — it can also power data sharing solutions to facilitate cooperation across departments or companies. Its potential comes from the ability to provide guarantees automatically without lengthy, burdensome privacy risk assessments.
Projects used to require a custom risk analysis and a custom data masking strategy to implement into the data pipeline pipeline. Differential privacy takes the onus off compliance teams, letting math and computers algorithmically determine how to protect user privacy cheaply, quickly and reliably.
The shift to differential privacy will be critical for companies looking to stay nimble as they capture part of what McKinsey estimates is $3 trillion of value generated by data collaboration.
Comment